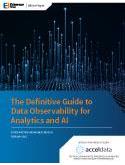
Data teams struggle to architect, build, and operate data systems to meet rapidly expanding business requirements. Data observability seeks to address this new world of unprecedented data complexity with a systematic approach that builds on its predecessor technology, application performance monitoring (APM). It monitors and correlates data events across the data pipeline, data, and infrastructure layers, enabling business owners, DevOps engineers, data architects, data engineers and site reliability engineers to detect, predict, prevent, and resolve issues—in an automated fashion—that would otherwise break production analytics and AI. To succeed with data observability, data analytics leaders must assemble and prioritize requirements, then select a comprehensive data observability product that minimizes custom integration work. They should tackle small, achievable observability projects first, enlisting a cross-functional team of contributors to focus on key pain points, such as performance and efficiency. Success on early projects can lead to more ambitious observability efforts—provided business and IT leaders continue to replace and retire duplicative older tools.