A Guide to Better Survivorship
The importance of survivorship, known as the "Golden Record" in data terms, is quite often overlooked in the quest for clean and effective customer data. Yet this final step in the record matching and consolidation process is more important than ever – in business today, it ultimately allows for the creation of a single, accurate and complete version of a customer record.
Even if you have invested in a state-of-the-art matching tool, and through careful analysis have constructed a matching policy that will catch all the duplicates in your database – how do you determine the most accurate data to use in establishing the Golden Record? Applying intelligent rules based on reference data – rather than just using the most recent record – is a new approach, and one that is increasing the value of Golden Record data.
Golden Record Basics
After running the matching process, you may be presented with the duplicated records bundled nicely into duplicate groups and ready for consolidation. Obvious matches such John Smith at 123 Main St and John Smythe at 123 Mein Street, are both identified as having the same information. Now what comes next? What do you do with the duplicates once they are detected?
In the matching methodology, choosing the unique or winning Golden Record is the next logical step. The process of selecting surviving records means selecting the best possible candidate as its representation. However, best in the perspective of survivorship can really mean a lot of things. It can be affected by the structure of data, the source of the data, how the data is populated, what kind of data is stored, and sometimes by the nature of business rules. Thus techniques can be applied in order to accommodate certain types of variations when performing survivorship.
Traditional Survivorship Techniques
Which record do we keep as our survivor and which ones do we discard? There are three commonly used techniques in determining the surviving record. In the Most Recent methodology, date-stamped records can be ordered from most recent to least recent. The most recent record can be considered eligible as the survivor. The Most Frequent approach matches records containing the same information as an indication of their correctness. Repeating records indicate that the information is persistent and therefore reliable. Finally, the Most Complete method considers field completeness as its primary factor of correctness. Records with more values populated for each available field are considered the most viable candidates for survivorship.
Although these techniques are commonly applied in survivorship schemas, correctness may not be as reliable in many circumstances. Because these techniques apply to almost any type of data, the basis on which a surviving record is created conforms only to “generic” rules. In contrast, by leveraging reference data, database administrators (DBAs) can generate better and more effective schemas for survivorship.
Evolving to Reference Data
Applying reference data in survivorship changes how rules come into play. Using the Most Recent, Most Frequent or Most Complete logic really has more of an aesthetic basis for selection. Ideally, the selection of the surviving record should be based on an actual understanding of data.
And this is where reference data has impact. Most importantly, it focuses solely on being able to consolidate the best quality data. By incorporating reference data, DBAs gain an understanding of the actual contents of data, and create better decisions for survivorship. Changing the perspective as to how the quality of data is defined in turn breaks the norm of typical survivorship schemas.
Survivorship Decisions
Let’s take a look at some instances involving how reference data and data quality affect decisions for survivorship.
I. Address Quality
Address quality is essential, and separating good data from bad data should take precedence in survivorship decisions. In the case of addresses, giving priority to good addresses makes for a better decision in the survivorship schema as opposed to selecting the most frequent.
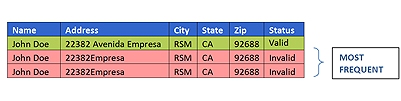
II. Record Quality
It could also be argued that good data may exist in a single group of matching records. In cases like these, we can assess the overall quality of data by taking into consideration other pieces of information that affect the weight of overall data quality. Take for example the following data:
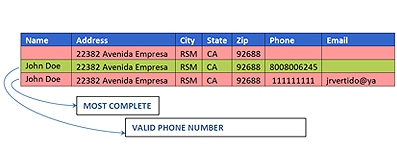
In this case, the ideal approach is to evaluate multiple elements for each record in the group. Since the second record contains a valid phone number, it can be given more weight or more importance than the third record, despite the third being more complete.
These images illustrate that the methodologies and logic used for record survivorship become dependent primarily on data quality, whether we’re working with contact data, product data or any other form of data. The focus on data quality transcends and even overrules other determining factors, such as which record was most complete or latest. For example:

It can be argued that the second record is the most recent one, and should therefore be the survivor. But upon careful consideration of the quality of the data, we can see that the second record contains an invalid phone number. This type of intelligent approach enables businesses with a more human perspective, and allows them to correctly conclude that the first record has the better data and should therefore be established as the Golden Record.
Applying New Perspective
However, we choose to define data quality, it is imperative that we keep only the best pieces of data if we are to have the most accurate and correct information. The most powerful future for data quality lies in our new and unique ability to discern contact data quality information and select the surviving record based on level of quality of the information provided.
This new technique for Golden Record selection offers a much more effective and logical approach when it comes to record survivorship. Ultimately, this creates an automatable system that can make smarter and better decisions for data cleansing – creating a single, accurate, high-value version of the truth that actually makes business sense.
About the author
Joseph Vertido, Data Quality Analyst, Melissa Data
Melissa Data recently introduced new Golden Record functionality in its MatchUp Component for SQL Server Integration Services (SSIS). A powerful tool for advanced matching and duplication management, specifically in the contact record domain, MatchUp SSIS dramatically enhances database efficiency and effectiveness by enabling a single, accurate view of each customer. By integrating the Golden Record selection tool, MatchUp SSIS represents an industry breakthrough based on its ability to discern contact data quality information, and select the surviving record based on the level of quality of the information provided.